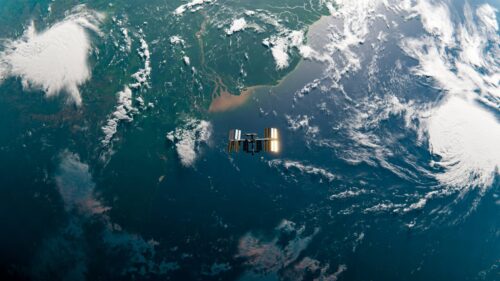
Can We Count on Forest Carbon Credits?
Technologies including remote sensing and machine learning have a critical role to play in enhancing the quality of forest carbon credits and building trust in carbon credit supply.
The voluntary carbon market (VCM) has a critical role to play in reducing and removing emissions from the atmosphere — but only if it can overcome widespread skepticism and accusations of greenwashing. On the supply side, the market faces mistrust around the quality of carbon credits. On the demand side, many are questioning whether large corporations — including those in the fossil fuel industry — are using carbon credits to avoid reducing their own emissions.
How can we bring trust to the VCM? In this article, we unpack one approach: leveraging technologies such as remote sensing and machine learning to improve the measurement and modeling of carbon quantities, and therefore the quality of carbon credits. Specifically, we focus on measurement and modeling in forestry — a sector that accounts for the largest share of VCM credits but has historically faced some of the biggest measurement and monitoring challenges.
Based on 30-plus expert interviews, this article describes how remote sensing and machine learning technologies are being used to model and monitor forest carbon quantities. The article addresses three key questions:
1) What problems in the carbon markets do these technologies solve?
2) Who is advancing technology solutions to these problems?
3) How can we accelerate the climate impact of these technologies?
Why Do Forest Carbon Measurements Matter?
Forest carbon credits cumulatively account for 44 percent (or more than 680 million tons of CO2 equivalence) of credit issued on the VCM as of 2022, higher than any other project types. But market players have struggled to demonstrate that these credits correspond to real-world emissions reductions at the claimed scale.
Remote sensing and machine learning help address this problem by measuring and modeling two things: aboveground biomass and changes in forest cover. Carbon project developers and verifiers then use that data to calculate four factors that define carbon credit quality — baseline, additionality, leakage, and durability.
Let’s look at these use cases one at a time:
Baseline refers to emissions that would have occurred without the project — or, in other words, the reference level against which carbon credits are calculated. For forest projects, determining baseline requires calculating the biomass of trees. Historically, project developers and verifiers have done so via tedious field plot measurements — sending surveyors into forests to manually measure trees. The plot samples are then extrapolated as an average for the entire forest. New technologies reduce cost and boost accuracy by leveraging light detection and ranging (LiDAR) for sample collection in order to reconstruct forest structure (e.g., tree height, canopy volume). Machine learning models, trained on and calibrated by LiDAR and field plot data, can then scale up biomass estimation at low cost.
Additionality means that the emissions reductions or removals would not have occurred in the absence of the carbon credits. Historically, additionality has been assessed alongside baseline before the project starts, with the assumption that additionality does not change over the course of a project. However, we have seen that policy or market fluctuations can lead to projects no longer being additional and therefore being over-credited. Satellites provide continuous monitoring of forest cover changes, detecting patterns in deforestation, forest degradation, and forest restoration. These Earth observation technologies enable more frequent assessments and updates of baselines. These technologies also enable post-implementation assessments of additionality based on what happened on the ground.
Leakage occurs when a project causes an increase in emissions outside the project boundary (e.g., if protecting one area of forest results in increased logging in a nearby area). Defining the area assessed for leakage (known as the “leakage belt”) has proven challenging. To date, a wide range of methods and data sources have been used to calculate the leakage belt, including self-reporting. This level of subjectivity has made it difficult for developers to collect data and for third parties to verify leakage assessments. Satellites solve this challenge by providing consistent and independent data sources that can create unified methods for leakage assessment.
Durability (often used interchangeably with permanence) describes how long a project is expected to reduce or remove carbon, or the likelihood that the emissions reduction or removal will be reversed. Forests — which are vulnerable to wildfire, droughts, and diseases — have notoriously performed poorly on measurements of durability or permanence. To tackle this challenge, forest carbon project developers typically contribute credits to a “buffer pool” — a reserve of nontradable credits that covers unforeseen losses in carbon stocks. However, accounting for buffer credits has not kept pace with increased reversal risks. Recent wildfires eliminated almost all of the California carbon trading system’s buffer pool, which was intended to cover reversal risks for the next 100 years. Satellite data and climate risk modeling can help ensure buffer pools are frequently recalibrated to account for the impacts of worsening climate conditions.
Who Are the Key Actors?
Actors across the VCM value chain are collecting remote sensing data and incorporating machine learning into various services and products. We have observed five types of actors: data collectors, data processors, project developers, brokers or exchanges, and ratings agencies.
Data collectors originate data from a wide range of technologies. At a global level, space agencies are collecting data through space-borne technologies including optical satellites, synthetic aperture radar, and space-borne LiDAR. Commercial satellite companies provide higher-resolution satellite data for specific locations. And a number of private entities are further calibrating data sets through airborne and on-the-ground LiDAR and field plots. In some regions with established forestry monitoring systems, national and jurisdictional governments also collect LiDAR and field plots systematically to establish forest inventories.
Data processors convert raw remote sensing data into proprietary data products for forest carbon modeling and measurement, while some also contextualize the data for carbon credits. Those products include biomass maps, wildfire risk analysis, and land management tools.
Ratings agencies provide an additional layer of monitoring and verification beyond conventional auditing and sell ratings to buyers as due diligence services. Ratings are based on assessments of additionality, permanence, and baseline, with some rating agencies introducing innovative measurement approaches.
Project developers execute new classes of projects using new methodologies and data sets. High-resolution data sets can reduce verification costs, therefore enabling small-scale landowners to develop forestry projects for carbon credits.
Brokers and exchanges sell carbon credits whose quality is vetted by remote sensing and machine learning technologies. These actors offer a bridge between project developers and buyers seeking high-quality carbon credits.
Greater collaboration between these actors could prevent duplication of efforts, which we are currently seeing in the data collection and processing space.
How Can We Accelerate Technology Impact?
Over the past couple of years, we’ve seen an explosion of remote sensing and machine learning technologies on the VCM landscape. Data is collected but remains in silos of individual ownership rather than contributing to the market’s larger goal: scaling up technology solutions and accelerating their adoption. Taking technology solutions to the global scale requires collecting and structuring tremendous amounts of data, overcoming institutional barriers, and winning the support of existing actors. To achieve all of these goals, collaboration across various actors will be critical. Specifically, we see an opportunity for collaboration in three areas:
- Improving accuracy. LiDAR and machine learning have elevated accuracy for aboveground biomass measurements. Developing an open data repository and uniform quality control for machine learning algorithms are the next key opportunities for accelerating adoption and improving trust. An open-source repository that makes LiDAR and field plot data readily available will make machine learning models created for unique biomes and geographies more affordable — unlocking adoption at the global scale. Independent quality control audits can provide a trustworthy objective performance assessment. By running the results of their machine learning algorithms against a common set of well-understood test beds, providers can validate their proprietary models without making the algorithms themselves publicly accessible.
- Developing standards. Most products on the market focus on ex post risk assessments. This means a new project still needs to go through manual measurements for registration and verification. Some early movers are seeking to replace the costly manual measurements with tech-based approaches. There are two ways of doing this. One is to build tailored methodologies, and the other is to allow the technologies to be used for quantification and verification in existing methodologies (e.g., the ABACUS label under Verra). We hope this process will advance standardization and transparency of technologies by establishing data protocols, identifying best practices for calibration, and quantifying uncertainties and risks.
- Sharing knowledge. Despite great interest and motivation in understanding how various technologies can help build quality and trust in the VCM, there is a disconnect between the technology community, buyers and investors, and suppliers of forest carbon credits. Project developers and the validating and verifying bodies will need training and buyers will need guidance on how to interpret the results and uncertainties.
In line with RMI’s “think, do, scale” approach, the Carbon Markets Initiative will facilitate a consortium of stakeholders to encourage collaboration within and beyond the VCM. RMI will continue to advocate for greater transparency and visibility of cutting-edge, technology-enabled solutions in forest carbon markets. We look forward to working with project developers, standard setters and governance bodies, and frontline communities to better integrate new technologies into the existing market infrastructure of the VCM to scale up their impact.